注意
转到末尾 下载完整示例代码。
使用 ONNX 算子¶
ONNX 旨在描述 scikit-learn 中实现的大多数机器学习模型,但它并不一定以与 scikit-learn 相同的方式描述预测函数。虽然可以定义自定义算子,但通常需要一些时间才能将其添加到 ONNX 规范中,然后添加到用于计算预测的后端。最好先查看现有的算子是否可以使用。该列表在 github 上提供,并提供了 基本算子 和其他 专用于机器学习的算子。ONNX 具有 Python API,可用于定义 ONNX 图:PythonAPIOverview.md。但它非常冗长,使得描述大型图变得困难。sklearn-onnx 实现了一种更方便的方式来测试 ONNX 算子。
ONNX Python API¶
让我们尝试 ONNX 文档中给出的示例:ONNX Model Using Helper Functions。它依赖于 protobuf,其定义可在 github 上找到 onnx.proto。
import onnxruntime
import numpy
import os
import numpy as np
import matplotlib.pyplot as plt
import onnx
from onnx import helper
from onnx import TensorProto
from onnx.tools.net_drawer import GetPydotGraph, GetOpNodeProducer
# Create one input (ValueInfoProto)
X = helper.make_tensor_value_info("X", TensorProto.FLOAT, [None, 2])
# Create one output (ValueInfoProto)
Y = helper.make_tensor_value_info("Y", TensorProto.FLOAT, [None, 4])
# Create a node (NodeProto)
node_def = helper.make_node(
"Pad", # node name
["X"], # inputs
["Y"], # outputs
mode="constant", # attributes
value=1.5,
pads=[0, 1, 0, 1],
)
# Create the graph (GraphProto)
graph_def = helper.make_graph(
[node_def],
"test-model",
[X],
[Y],
)
# Create the model (ModelProto)
model_def = helper.make_model(graph_def, producer_name="onnx-example")
model_def.opset_import[0].version = 10
print("The model is:\n{}".format(model_def))
onnx.checker.check_model(model_def)
print("The model is checked!")
The model is:
ir_version: 10
opset_import {
version: 10
}
producer_name: "onnx-example"
graph {
node {
input: "X"
output: "Y"
op_type: "Pad"
attribute {
name: "mode"
type: STRING
s: "constant"
}
attribute {
name: "pads"
type: INTS
ints: 0
ints: 1
ints: 0
ints: 1
}
attribute {
name: "value"
type: FLOAT
f: 1.5
}
}
name: "test-model"
input {
name: "X"
type {
tensor_type {
elem_type: 1
shape {
dim {
}
dim {
dim_value: 2
}
}
}
}
}
output {
name: "Y"
type {
tensor_type {
elem_type: 1
shape {
dim {
}
dim {
dim_value: 4
}
}
}
}
}
}
The model is checked!
与 sklearn-onnx 相同的示例¶
每个算子在 sklearn-onnx 中都有自己的类。该列表是根据已安装的 onnx 包动态创建的。
from skl2onnx.algebra.onnx_ops import OnnxPad # noqa
pad = OnnxPad(
"X",
output_names=["Y"],
mode="constant",
value=1.5,
pads=[0, 1, 0, 1],
op_version=10,
)
model_def = pad.to_onnx({"X": X}, target_opset=10)
print("The model is:\n{}".format(model_def))
onnx.checker.check_model(model_def)
print("The model is checked!")
The model is:
ir_version: 5
opset_import {
domain: ""
version: 10
}
producer_name: "skl2onnx"
producer_version: "1.17.0"
domain: "ai.onnx"
model_version: 0
graph {
node {
input: "X"
output: "Y"
name: "Pa_Pad"
op_type: "Pad"
domain: ""
attribute {
name: "mode"
type: STRING
s: "constant"
}
attribute {
name: "pads"
type: INTS
ints: 0
ints: 1
ints: 0
ints: 1
}
attribute {
name: "value"
type: FLOAT
f: 1.5
}
}
name: "OnnxPad"
input {
name: "X"
type {
tensor_type {
elem_type: 1
shape {
dim {
}
dim {
dim_value: 2
}
}
}
}
}
output {
name: "Y"
type {
tensor_type {
elem_type: 1
shape {
dim {
}
dim {
dim_value: 4
}
}
}
}
}
}
The model is checked!
输入和输出也可以跳过。
pad = OnnxPad(mode="constant", value=1.5, pads=[0, 1, 0, 1], op_version=10)
model_def = pad.to_onnx({pad.inputs[0].name: X}, target_opset=10)
onnx.checker.check_model(model_def)
多个算子¶
让我们使用文档中的第二个示例。
# Preprocessing: create a model with two nodes, Y's shape is unknown
node1 = helper.make_node("Transpose", ["X"], ["Y"], perm=[1, 0, 2])
node2 = helper.make_node("Transpose", ["Y"], ["Z"], perm=[1, 0, 2])
graph = helper.make_graph(
[node1, node2],
"two-transposes",
[helper.make_tensor_value_info("X", TensorProto.FLOAT, (2, 3, 4))],
[helper.make_tensor_value_info("Z", TensorProto.FLOAT, (2, 3, 4))],
)
original_model = helper.make_model(graph, producer_name="onnx-examples")
# Check the model and print Y's shape information
onnx.checker.check_model(original_model)
我们将其转换为
from skl2onnx.algebra.onnx_ops import OnnxTranspose # noqa
node = OnnxTranspose(
OnnxTranspose("X", perm=[1, 0, 2], op_version=12), perm=[1, 0, 2], op_version=12
)
X = np.arange(2 * 3 * 4).reshape((2, 3, 4)).astype(np.float32)
# numpy arrays are good enough to define the input shape
model_def = node.to_onnx({"X": X}, target_opset=12)
onnx.checker.check_model(model_def)
让我们使用 onnxruntime 输出结果
def predict_with_onnxruntime(model_def, *inputs):
import onnxruntime as ort
sess = ort.InferenceSession(
model_def.SerializeToString(), providers=["CPUExecutionProvider"]
)
names = [i.name for i in sess.get_inputs()]
dinputs = {name: input for name, input in zip(names, inputs)}
res = sess.run(None, dinputs)
names = [o.name for o in sess.get_outputs()]
return {name: output for name, output in zip(names, res)}
Y = predict_with_onnxruntime(model_def, X)
print(Y)
{'Tr_transposed0': array([[[ 0., 1., 2., 3.],
[ 4., 5., 6., 7.],
[ 8., 9., 10., 11.]],
[[12., 13., 14., 15.],
[16., 17., 18., 19.],
[20., 21., 22., 23.]]], dtype=float32)}
显示 ONNX 图¶
pydot_graph = GetPydotGraph(
model_def.graph,
name=model_def.graph.name,
rankdir="TB",
node_producer=GetOpNodeProducer(
"docstring", color="yellow", fillcolor="yellow", style="filled"
),
)
pydot_graph.write_dot("pipeline_transpose2x.dot")
os.system("dot -O -Gdpi=300 -Tpng pipeline_transpose2x.dot")
image = plt.imread("pipeline_transpose2x.dot.png")
fig, ax = plt.subplots(figsize=(40, 20))
ax.imshow(image)
ax.axis("off")
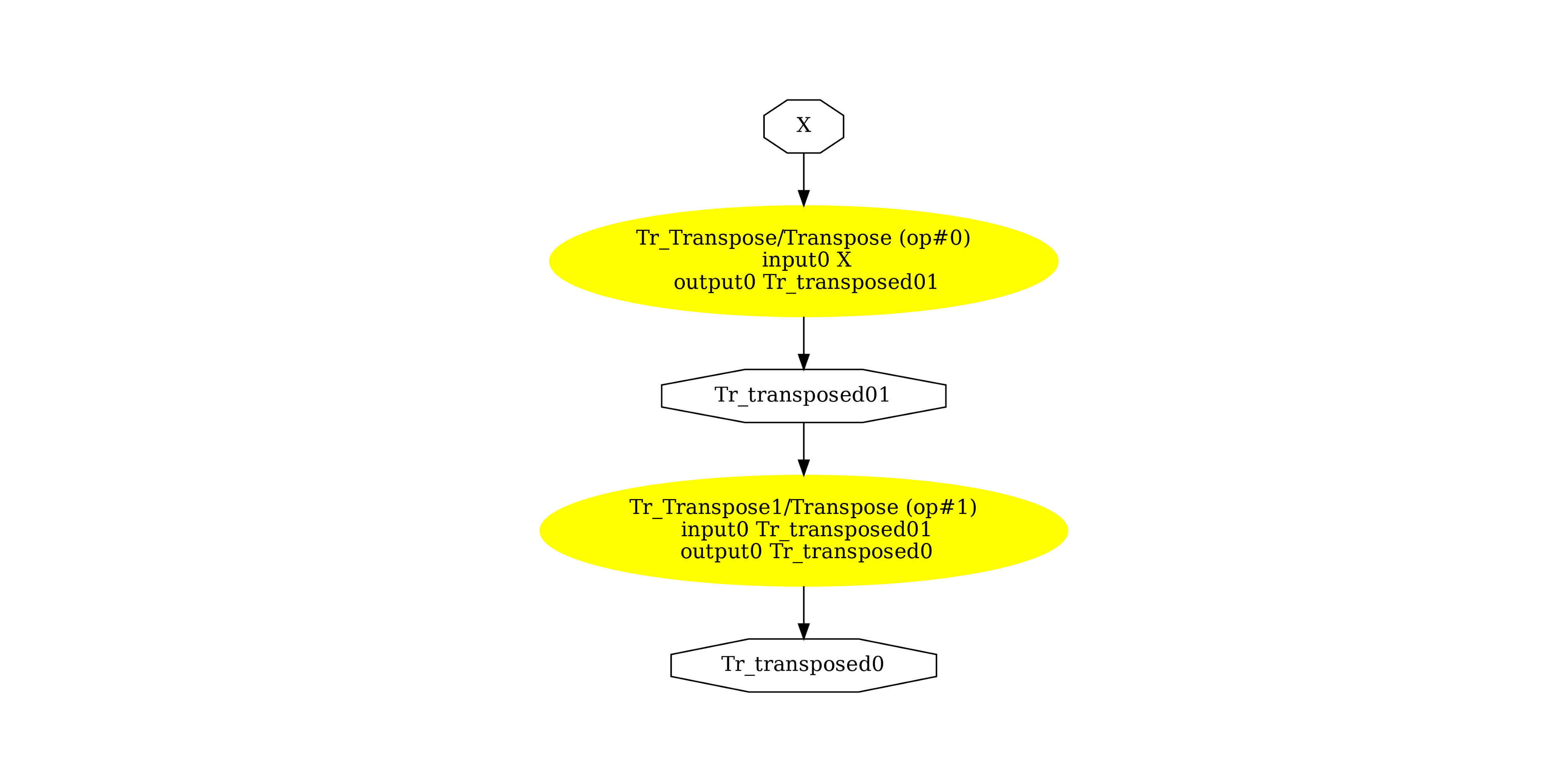
(-0.5, 1524.5, 1707.5, -0.5)
此示例使用的版本
import sklearn # noqa
print("numpy:", numpy.__version__)
print("scikit-learn:", sklearn.__version__)
import skl2onnx # noqa
print("onnx: ", onnx.__version__)
print("onnxruntime: ", onnxruntime.__version__)
print("skl2onnx: ", skl2onnx.__version__)
numpy: 1.26.4
scikit-learn: 1.6.dev0
onnx: 1.17.0
onnxruntime: 1.18.0+cu118
skl2onnx: 1.17.0
脚本总运行时间:(0 分钟 0.882 秒)