注意
转到末尾 下载完整的示例代码。
遍历中间输出¶
我们重新使用示例 将带有 ColumnTransformer 的管道进行转换 并遍历中间输出。 转换后的模型很有可能产生不同的输出或由于未正确实现的自定义转换器而失败。 一个选项是查看 ONNX 图中每个节点的输出。
创建和训练复杂管道¶
我们重新使用在示例 带有混合类型的 Column Transformer 中实现的管道。 由于 ONNX-ML Imputer 不处理字符串类型,因此存在一个变化。 这不能是最终 ONNX 管道的一部分,必须删除。 查找以下以 ---
开头的注释。
import skl2onnx
import onnx
import sklearn
import matplotlib.pyplot as plt
import os
from onnx.tools.net_drawer import GetPydotGraph, GetOpNodeProducer
from skl2onnx.helpers.onnx_helper import select_model_inputs_outputs
from skl2onnx.helpers.onnx_helper import save_onnx_model
from skl2onnx.helpers.onnx_helper import enumerate_model_node_outputs
from skl2onnx.helpers.onnx_helper import load_onnx_model
import numpy
import onnxruntime as rt
from skl2onnx import convert_sklearn
import pprint
from skl2onnx.common.data_types import (
FloatTensorType,
StringTensorType,
Int64TensorType,
)
import numpy as np
import pandas as pd
from sklearn.compose import ColumnTransformer
from sklearn.pipeline import Pipeline
from sklearn.impute import SimpleImputer
from sklearn.preprocessing import StandardScaler, OneHotEncoder
from sklearn.linear_model import LogisticRegression
from sklearn.model_selection import train_test_split
titanic_url = (
"https://raw.githubusercontent.com/amueller/"
"scipy-2017-sklearn/091d371/notebooks/datasets/titanic3.csv"
)
data = pd.read_csv(titanic_url)
X = data.drop("survived", axis=1)
y = data["survived"]
# SimpleImputer on string is not available
# for string in ONNX-ML specifications.
# So we do it beforehand.
for cat in ["embarked", "sex", "pclass"]:
X[cat].fillna("missing", inplace=True)
X_train, X_test, y_train, y_test = train_test_split(X, y, test_size=0.2)
numeric_features = ["age", "fare"]
numeric_transformer = Pipeline(
steps=[("imputer", SimpleImputer(strategy="median")), ("scaler", StandardScaler())]
)
categorical_features = ["embarked", "sex", "pclass"]
categorical_transformer = Pipeline(
steps=[
# --- SimpleImputer is not available for strings in ONNX-ML specifications.
# ('imputer', SimpleImputer(strategy='constant', fill_value='missing')),
("onehot", OneHotEncoder(handle_unknown="ignore"))
]
)
preprocessor = ColumnTransformer(
transformers=[
("num", numeric_transformer, numeric_features),
("cat", categorical_transformer, categorical_features),
]
)
clf = Pipeline(
steps=[
("preprocessor", preprocessor),
("classifier", LogisticRegression(solver="lbfgs")),
]
)
clf.fit(X_train, y_train)
定义 ONNX 图的输入¶
sklearn-onnx 不知道用于训练模型的特征,但它需要知道哪个特征具有哪个名称。 我们只需重新使用数据框的列定义。
print(X_train.dtypes)
pclass int64
name object
sex object
age float64
sibsp int64
parch int64
ticket object
fare float64
cabin object
embarked object
boat object
body float64
home.dest object
dtype: object
转换后。
def convert_dataframe_schema(df, drop=None):
inputs = []
for k, v in zip(df.columns, df.dtypes):
if drop is not None and k in drop:
continue
if v == "int64":
t = Int64TensorType([None, 1])
elif v == "float64":
t = FloatTensorType([None, 1])
else:
t = StringTensorType([None, 1])
inputs.append((k, t))
return inputs
inputs = convert_dataframe_schema(X_train)
pprint.pprint(inputs)
[('pclass', Int64TensorType(shape=[None, 1])),
('name', StringTensorType(shape=[None, 1])),
('sex', StringTensorType(shape=[None, 1])),
('age', FloatTensorType(shape=[None, 1])),
('sibsp', Int64TensorType(shape=[None, 1])),
('parch', Int64TensorType(shape=[None, 1])),
('ticket', StringTensorType(shape=[None, 1])),
('fare', FloatTensorType(shape=[None, 1])),
('cabin', StringTensorType(shape=[None, 1])),
('embarked', StringTensorType(shape=[None, 1])),
('boat', StringTensorType(shape=[None, 1])),
('body', FloatTensorType(shape=[None, 1])),
('home.dest', StringTensorType(shape=[None, 1]))]
将单个列合并成向量不是计算预测的最高效方法。 这可以在将管道转换为图形之前完成。
将管道转换为 ONNX¶
try:
model_onnx = convert_sklearn(clf, "pipeline_titanic", inputs, target_opset=12)
except Exception as e:
print(e)
scikit-learn 在可以的情况下执行隐式转换。 sklearn-onnx 不会。 OneHotEncoder 的 ONNX 版本必须应用于相同类型的列。
X_train["pclass"] = X_train["pclass"].astype(str)
X_test["pclass"] = X_test["pclass"].astype(str)
white_list = numeric_features + categorical_features
to_drop = [c for c in X_train.columns if c not in white_list]
inputs = convert_dataframe_schema(X_train, to_drop)
model_onnx = convert_sklearn(clf, "pipeline_titanic", inputs, target_opset=12)
# And save.
with open("pipeline_titanic.onnx", "wb") as f:
f.write(model_onnx.SerializeToString())
比较预测¶
最后一步,我们需要确保转换后的模型产生相同的预测、标签和概率。 让我们从 scikit-learn 开始。
predict [0 0 1 0 0]
predict_proba [[0.60224126 0.39775874]]
使用 onnxruntime 的预测。 我们需要删除已删除的列并将双向量更改为浮点向量,因为 onnxruntime 不支持双精度浮点数。 onnxruntime 不接受 数据框。 输入必须作为字典列表给出。 最后,每个列都被描述为不是真正的向量,而是具有单列的矩阵,这解释了最后一行的 reshape 操作。
我们已准备好运行 onnxruntime。
predict [0 0 1 0 0]
predict_proba [{0: 0.7899309396743774, 1: 0.21006903052330017}]
计算中间输出¶
不幸的是,实际上没有办法让 onnxruntime 检索中间节点的输出。 我们需要修改 ONNX,然后再将其提供给 onnxruntime。 让我们首先查看中间输出的列表。
merged_columns
embarkedout
sexout
pclassout
concat_result
variable
variable2
variable1
transformed_column
label
probabilities
output_label
output_probability
很难判断哪个是哪个,因为 ONNX 拥有比原始 scikit-learn 管道更多的操作符。 显示 ONNX 图 中的图有助于我们找到数值和文本管道的输出:variable1、variable2。 让我们先看看数值管道。
num_onnx = select_model_inputs_outputs(model_onnx, "variable1")
save_onnx_model(num_onnx, "pipeline_titanic_numerical.onnx")
b'\x08\x07\x12\x08skl2onnx\x1a\x061.17.0"\x07ai.onnx(\x002\x00:\xcd\x03\n:\n\x03age\n\x04fare\x12\x0emerged_columns\x1a\x06Concat"\x06Concat*\x0b\n\x04axis\x18\x01\xa0\x01\x02:\x00\n}\n\x0emerged_columns\x12\x08variable\x1a\x07Imputer"\x07Imputer*#\n\x14imputed_value_floats=\x00\x00\xe2A=\xcdLgA\xa0\x01\x06*\x1e\n\x14replaced_value_float\x15\x00\x00\xc0\x7f\xa0\x01\x01:\nai.onnx.ml\n^\n\x08variable\x12\tvariable1\x1a\x06Scaler"\x06Scaler*\x15\n\x06offset=\xe05\xedA=\'\xcb\nB\xa0\x01\x06*\x14\n\x05scale=\'l\x9f==\xdd,\x96<\xa0\x01\x06:\nai.onnx.ml\x12\x10pipeline_titanic*\x1f\x08\x02\x10\x07:\x0b\xff\xff\xff\xff\xff\xff\xff\xff\xff\x01\tB\x0cshape_tensorZ\x16\n\x06pclass\x12\x0c\n\n\x08\x08\x12\x06\n\x00\n\x02\x08\x01Z\x13\n\x03sex\x12\x0c\n\n\x08\x08\x12\x06\n\x00\n\x02\x08\x01Z\x13\n\x03age\x12\x0c\n\n\x08\x01\x12\x06\n\x00\n\x02\x08\x01Z\x14\n\x04fare\x12\x0c\n\n\x08\x01\x12\x06\n\x00\n\x02\x08\x01Z\x18\n\x08embarked\x12\x0c\n\n\x08\x08\x12\x06\n\x00\n\x02\x08\x01b\x0b\n\tvariable1B\x0e\n\nai.onnx.ml\x10\x01B\x04\n\x00\x10\x0b'
让我们计算数值特征。
numerical features [[-0.7512866 -0.50364053]]
对于文本特征,我们执行相同的操作。
print(model_onnx)
text_onnx = select_model_inputs_outputs(model_onnx, "variable2")
save_onnx_model(text_onnx, "pipeline_titanic_textual.onnx")
sess = rt.InferenceSession(
"pipeline_titanic_textual.onnx", providers=["CPUExecutionProvider"]
)
numT = sess.run(None, inputs)
print("textual features", numT[0][:1])
ir_version: 7
opset_import {
domain: "ai.onnx.ml"
version: 1
}
opset_import {
domain: ""
version: 11
}
producer_name: "skl2onnx"
producer_version: "1.17.0"
domain: "ai.onnx"
model_version: 0
doc_string: ""
graph {
node {
input: "age"
input: "fare"
output: "merged_columns"
name: "Concat"
op_type: "Concat"
domain: ""
attribute {
name: "axis"
type: INT
i: 1
}
}
node {
input: "embarked"
output: "embarkedout"
name: "OneHotEncoder"
op_type: "OneHotEncoder"
domain: "ai.onnx.ml"
attribute {
name: "cats_strings"
type: STRINGS
strings: "C"
strings: "Q"
strings: "S"
strings: "missing"
}
attribute {
name: "zeros"
type: INT
i: 1
}
}
node {
input: "sex"
output: "sexout"
name: "OneHotEncoder1"
op_type: "OneHotEncoder"
domain: "ai.onnx.ml"
attribute {
name: "cats_strings"
type: STRINGS
strings: "female"
strings: "male"
}
attribute {
name: "zeros"
type: INT
i: 1
}
}
node {
input: "pclass"
output: "pclassout"
name: "OneHotEncoder2"
op_type: "OneHotEncoder"
domain: "ai.onnx.ml"
attribute {
name: "cats_strings"
type: STRINGS
strings: "1"
strings: "2"
strings: "3"
}
attribute {
name: "zeros"
type: INT
i: 1
}
}
node {
input: "embarkedout"
input: "sexout"
input: "pclassout"
output: "concat_result"
name: "Concat1"
op_type: "Concat"
domain: ""
attribute {
name: "axis"
type: INT
i: -1
}
}
node {
input: "merged_columns"
output: "variable"
name: "Imputer"
op_type: "Imputer"
domain: "ai.onnx.ml"
attribute {
name: "imputed_value_floats"
type: FLOATS
floats: 28.25
floats: 14.4562502
}
attribute {
name: "replaced_value_float"
type: FLOAT
f: nan
}
}
node {
input: "concat_result"
input: "shape_tensor"
output: "variable2"
name: "Reshape"
op_type: "Reshape"
domain: ""
}
node {
input: "variable"
output: "variable1"
name: "Scaler"
op_type: "Scaler"
domain: "ai.onnx.ml"
attribute {
name: "offset"
type: FLOATS
floats: 29.6513062
floats: 34.698391
}
attribute {
name: "scale"
type: FLOATS
floats: 0.077843
floats: 0.0183319394
}
}
node {
input: "variable1"
input: "variable2"
output: "transformed_column"
name: "Concat2"
op_type: "Concat"
domain: ""
attribute {
name: "axis"
type: INT
i: 1
}
}
node {
input: "transformed_column"
output: "label"
output: "probabilities"
name: "LinearClassifier"
op_type: "LinearClassifier"
domain: "ai.onnx.ml"
attribute {
name: "classlabels_ints"
type: INTS
ints: 0
ints: 1
}
attribute {
name: "coefficients"
type: FLOATS
floats: 0.411349356
floats: -0.0257858913
floats: -0.341414243
floats: 0.0805286616
floats: 0.334271878
floats: -0.121588431
floats: -1.24841082
floats: 1.20020878
floats: -0.920275748
floats: -0.037623141
floats: 0.909696758
floats: -0.411349356
floats: 0.0257858913
floats: 0.341414243
floats: -0.0805286616
floats: -0.334271878
floats: 0.121588431
floats: 1.24841082
floats: -1.20020878
floats: 0.920275748
floats: 0.037623141
floats: -0.909696758
}
attribute {
name: "intercepts"
type: FLOATS
floats: -0.147927582
floats: 0.147927582
}
attribute {
name: "multi_class"
type: INT
i: 0
}
attribute {
name: "post_transform"
type: STRING
s: "LOGISTIC"
}
}
node {
input: "label"
output: "output_label"
name: "Cast"
op_type: "Cast"
domain: ""
attribute {
name: "to"
type: INT
i: 7
}
}
node {
input: "probabilities"
output: "output_probability"
name: "ZipMap"
op_type: "ZipMap"
domain: "ai.onnx.ml"
attribute {
name: "classlabels_int64s"
type: INTS
ints: 0
ints: 1
}
}
name: "pipeline_titanic"
initializer {
dims: 2
data_type: 7
int64_data: -1
int64_data: 9
name: "shape_tensor"
}
input {
name: "pclass"
type {
tensor_type {
elem_type: 8
shape {
dim {
}
dim {
dim_value: 1
}
}
}
}
}
input {
name: "sex"
type {
tensor_type {
elem_type: 8
shape {
dim {
}
dim {
dim_value: 1
}
}
}
}
}
input {
name: "age"
type {
tensor_type {
elem_type: 1
shape {
dim {
}
dim {
dim_value: 1
}
}
}
}
}
input {
name: "fare"
type {
tensor_type {
elem_type: 1
shape {
dim {
}
dim {
dim_value: 1
}
}
}
}
}
input {
name: "embarked"
type {
tensor_type {
elem_type: 8
shape {
dim {
}
dim {
dim_value: 1
}
}
}
}
}
output {
name: "output_label"
type {
tensor_type {
elem_type: 7
shape {
dim {
}
}
}
}
}
output {
name: "output_probability"
type {
sequence_type {
elem_type {
map_type {
key_type: 7
value_type {
tensor_type {
elem_type: 1
}
}
}
}
}
}
}
}
textual features [[1. 0. 0. 0. 0. 1. 0. 0. 1.]]
显示子 ONNX 图¶
最后,让我们看看两个子图。 首先是数值管道。
pydot_graph = GetPydotGraph(
num_onnx.graph,
name=num_onnx.graph.name,
rankdir="TB",
node_producer=GetOpNodeProducer(
"docstring", color="yellow", fillcolor="yellow", style="filled"
),
)
pydot_graph.write_dot("pipeline_titanic_num.dot")
os.system("dot -O -Gdpi=300 -Tpng pipeline_titanic_num.dot")
image = plt.imread("pipeline_titanic_num.dot.png")
fig, ax = plt.subplots(figsize=(40, 20))
ax.imshow(image)
ax.axis("off")
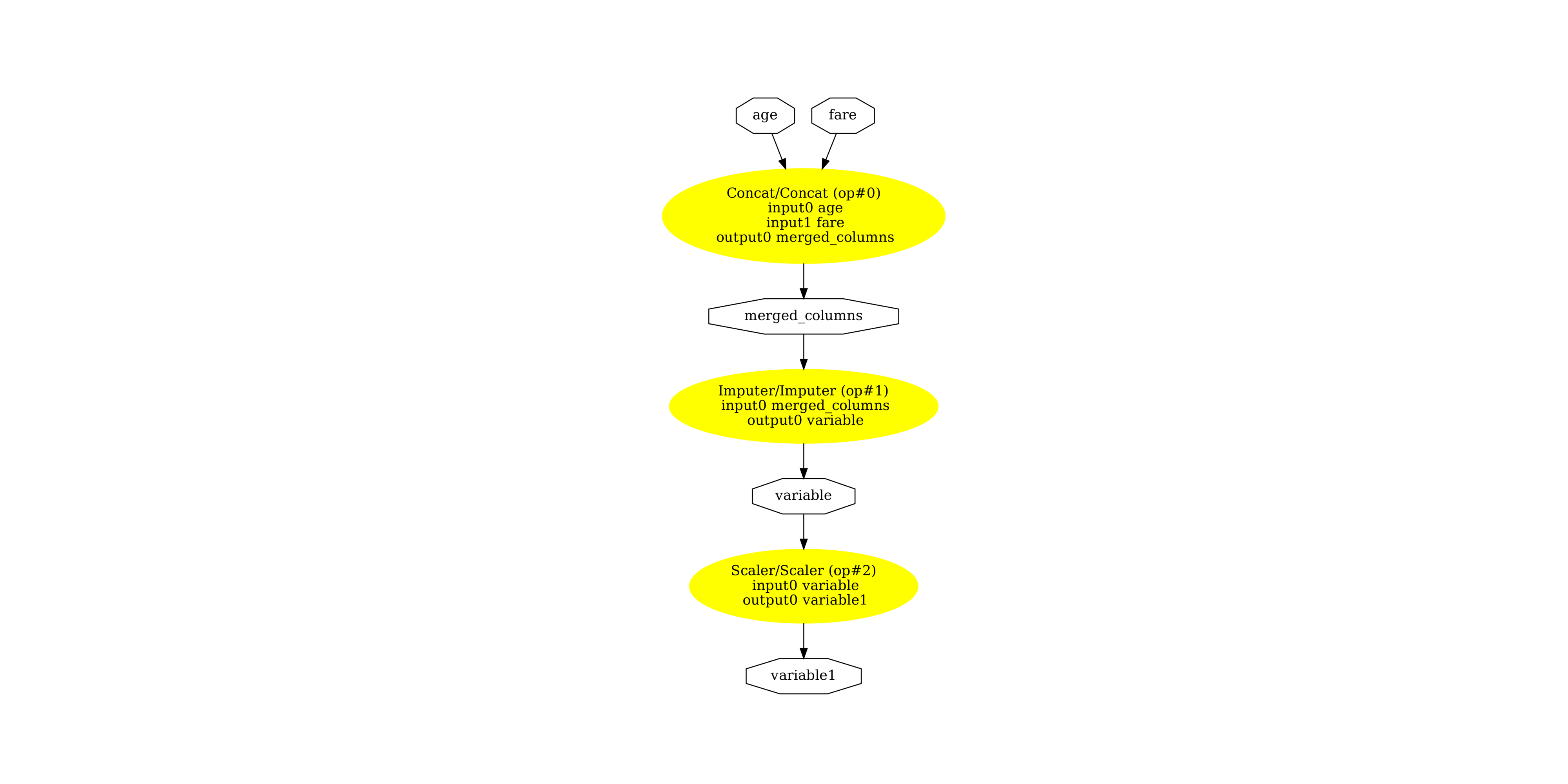
(-0.5, 1229.5, 2558.5, -0.5)
然后是文本管道。
pydot_graph = GetPydotGraph(
text_onnx.graph,
name=text_onnx.graph.name,
rankdir="TB",
node_producer=GetOpNodeProducer(
"docstring", color="yellow", fillcolor="yellow", style="filled"
),
)
pydot_graph.write_dot("pipeline_titanic_text.dot")
os.system("dot -O -Gdpi=300 -Tpng pipeline_titanic_text.dot")
image = plt.imread("pipeline_titanic_text.dot.png")
fig, ax = plt.subplots(figsize=(40, 20))
ax.imshow(image)
ax.axis("off")
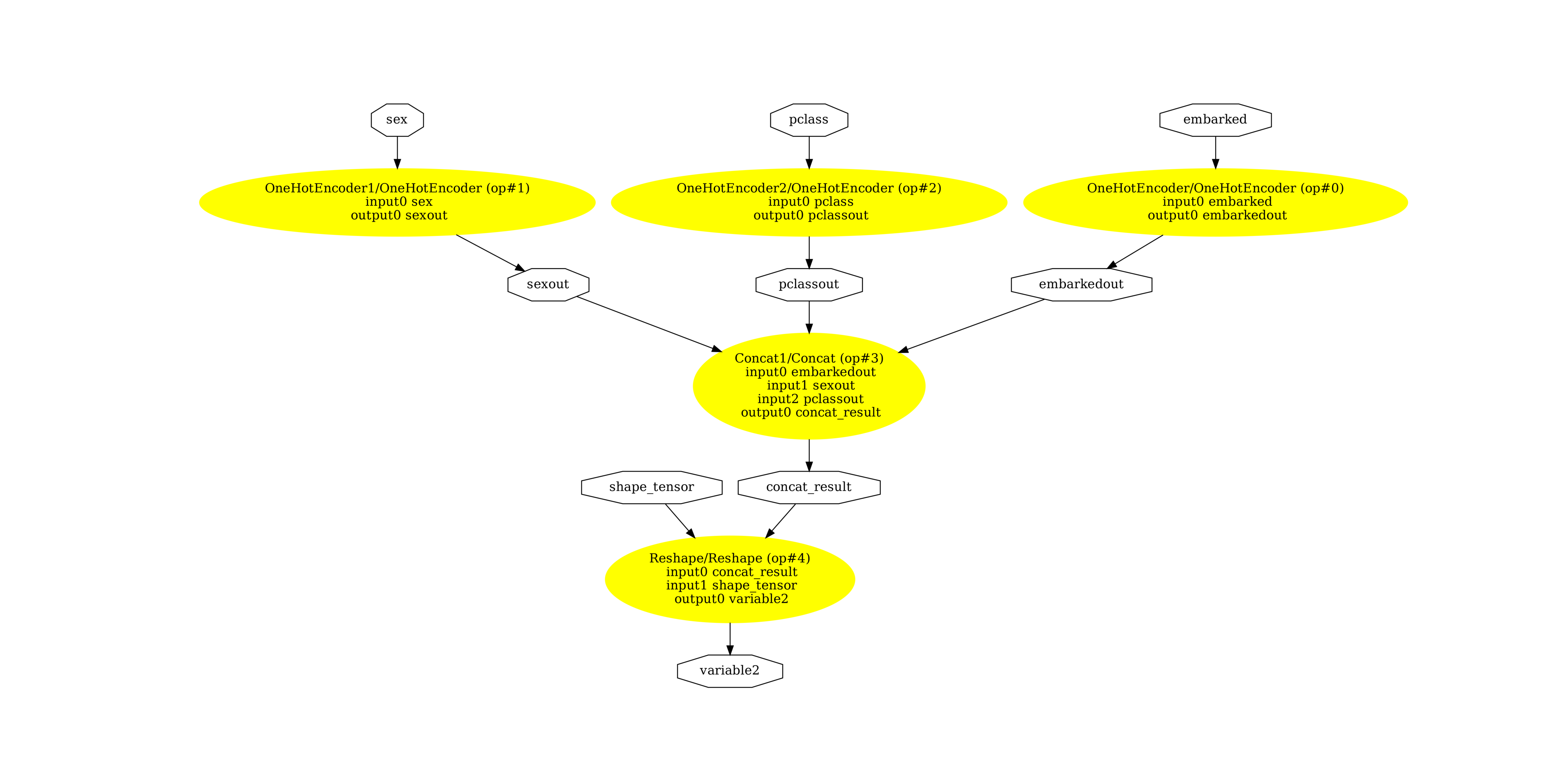
(-0.5, 5630.5, 2735.5, -0.5)
此示例使用的版本
print("numpy:", numpy.__version__)
print("scikit-learn:", sklearn.__version__)
print("onnx: ", onnx.__version__)
print("onnxruntime: ", rt.__version__)
print("skl2onnx: ", skl2onnx.__version__)
numpy: 1.26.4
scikit-learn: 1.6.dev0
onnx: 1.17.0
onnxruntime: 1.18.0+cu118
skl2onnx: 1.17.0
脚本总运行时间:(0 分钟 4.738 秒)