注意
转到末尾 下载完整示例代码。
转换带 ColumnTransformer 的管线¶
scikit-learn 最近推出了 ColumnTransformer,它允许用户定义复杂的管线,其中每一列都可以使用不同的转换器进行预处理。在这种情况下,sklearn-onnx 仍然有效,如 转换复杂管线 部分所示。
创建并训练一个复杂的管线¶
我们重用示例 混合类型列转换器 中实现的管线。有一个更改,因为 ONNX-ML Imputer 不处理字符串类型。这不能作为最终 ONNX 管线的一部分,必须被删除。请查看下面以 ---
开头的注释。
import os
import pprint
import pandas as pd
import numpy as np
from numpy.testing import assert_almost_equal
import onnx
from onnx.tools.net_drawer import GetPydotGraph, GetOpNodeProducer
import onnxruntime as rt
import matplotlib.pyplot as plt
import sklearn
from sklearn.compose import ColumnTransformer
from sklearn.pipeline import Pipeline
from sklearn.impute import SimpleImputer
from sklearn.preprocessing import StandardScaler, OneHotEncoder
from sklearn.linear_model import LogisticRegression
from sklearn.model_selection import train_test_split
import skl2onnx
from skl2onnx import convert_sklearn
from skl2onnx.common.data_types import FloatTensorType, StringTensorType
from skl2onnx.common.data_types import Int64TensorType
titanic_url = (
"https://raw.githubusercontent.com/amueller/"
"scipy-2017-sklearn/091d371/notebooks/datasets/titanic3.csv"
)
data = pd.read_csv(titanic_url)
X = data.drop("survived", axis=1)
y = data["survived"]
print(data.dtypes)
# SimpleImputer on string is not available for
# string in ONNX-ML specifications.
# So we do it beforehand.
for cat in ["embarked", "sex", "pclass"]:
X[cat].fillna("missing", inplace=True)
X_train, X_test, y_train, y_test = train_test_split(X, y, test_size=0.2)
numeric_features = ["age", "fare"]
numeric_transformer = Pipeline(
steps=[("imputer", SimpleImputer(strategy="median")), ("scaler", StandardScaler())]
)
categorical_features = ["embarked", "sex", "pclass"]
categorical_transformer = Pipeline(
steps=[
# --- SimpleImputer is not available for strings in ONNX-ML specifications.
# ('imputer', SimpleImputer(strategy='constant', fill_value='missing')),
("onehot", OneHotEncoder(handle_unknown="ignore"))
]
)
preprocessor = ColumnTransformer(
transformers=[
("num", numeric_transformer, numeric_features),
("cat", categorical_transformer, categorical_features),
]
)
clf = Pipeline(
steps=[
("preprocessor", preprocessor),
("classifier", LogisticRegression(solver="lbfgs")),
]
)
clf.fit(X_train, y_train)
pclass int64
survived int64
name object
sex object
age float64
sibsp int64
parch int64
ticket object
fare float64
cabin object
embarked object
boat object
body float64
home.dest object
dtype: object
定义 ONNX 图的输入¶
sklearn-onnx 不知道用于训练模型的特征,但它需要知道哪个特征有什么名称。我们只需重用 dataframe 的列定义。
print(X_train.dtypes)
pclass int64
name object
sex object
age float64
sibsp int64
parch int64
ticket object
fare float64
cabin object
embarked object
boat object
body float64
home.dest object
dtype: object
转换后。
def convert_dataframe_schema(df, drop=None):
inputs = []
for k, v in zip(df.columns, df.dtypes):
if drop is not None and k in drop:
continue
if v == "int64":
t = Int64TensorType([None, 1])
elif v == "float64":
t = FloatTensorType([None, 1])
else:
t = StringTensorType([None, 1])
inputs.append((k, t))
return inputs
initial_inputs = convert_dataframe_schema(X_train)
pprint.pprint(initial_inputs)
[('pclass', Int64TensorType(shape=[None, 1])),
('name', StringTensorType(shape=[None, 1])),
('sex', StringTensorType(shape=[None, 1])),
('age', FloatTensorType(shape=[None, 1])),
('sibsp', Int64TensorType(shape=[None, 1])),
('parch', Int64TensorType(shape=[None, 1])),
('ticket', StringTensorType(shape=[None, 1])),
('fare', FloatTensorType(shape=[None, 1])),
('cabin', StringTensorType(shape=[None, 1])),
('embarked', StringTensorType(shape=[None, 1])),
('boat', StringTensorType(shape=[None, 1])),
('body', FloatTensorType(shape=[None, 1])),
('home.dest', StringTensorType(shape=[None, 1]))]
将单列合并成向量并不是计算预测的最有效方法。这可以在将管线转换为图之前完成。
将管线转换为 ONNX¶
try:
model_onnx = convert_sklearn(
clf, "pipeline_titanic", initial_inputs, target_opset=12
)
except Exception as e:
print(e)
如果图很小,预测会更高效。这就是为什么转换器会检查是否存在未使用的输入。它们需要从图输入中移除。
to_drop = {"parch", "sibsp", "cabin", "ticket", "name", "body", "home.dest", "boat"}
initial_inputs = convert_dataframe_schema(X_train, to_drop)
try:
model_onnx = convert_sklearn(
clf, "pipeline_titanic", initial_inputs, target_opset=12
)
except Exception as e:
print(e)
scikit-learn 可以在可能的情况下进行隐式转换。sklearn-onnx 不会。ONNX 版本的 OneHotEncoder 必须应用于相同类型的列。
initial_inputs = convert_dataframe_schema(X_train, to_drop)
model_onnx = convert_sklearn(clf, "pipeline_titanic", initial_inputs, target_opset=12)
# And save.
with open("pipeline_titanic.onnx", "wb") as f:
f.write(model_onnx.SerializeToString())
比较预测结果¶
最后一步,我们需要确保转换后的模型产生相同的预测、标签和概率。我们从 scikit-learn 开始。
print("predict", clf.predict(X_test[:5]))
print("predict_proba", clf.predict_proba(X_test[:2]))
predict [1 1 1 0 0]
predict_proba [[0.45469426 0.54530574]
[0.27457194 0.72542806]]
使用 onnxruntime 进行预测。我们需要删除被丢弃的列,并将双精度向量更改为单精度向量,因为 onnxruntime 不支持双精度浮点数。onnxruntime 不接受 dataframe 作为输入,输入必须作为字典列表提供。最后一个细节是,每列都没有真正描述为向量,而是描述为单列矩阵,这解释了最后一行的 reshape。
X_test2 = X_test.drop(to_drop, axis=1)
inputs = {c: X_test2[c].values for c in X_test2.columns}
for c in numeric_features:
inputs[c] = inputs[c].astype(np.float32)
for k in inputs:
inputs[k] = inputs[k].reshape((inputs[k].shape[0], 1))
我们已经准备好运行 onnxruntime。
sess = rt.InferenceSession("pipeline_titanic.onnx", providers=["CPUExecutionProvider"])
pred_onx = sess.run(None, inputs)
print("predict", pred_onx[0][:5])
print("predict_proba", pred_onx[1][:2])
predict [1 1 1 0 0]
predict_proba [{0: 0.4546942710876465, 1: 0.5453057289123535}, {0: 0.27457195520401, 1: 0.72542804479599}]
onnxruntime 的输出是一个字典列表。让我们切换到数组,但这需要使用附加选项 zipmap 再次进行转换。
model_onnx = convert_sklearn(
clf,
"pipeline_titanic",
initial_inputs,
target_opset=12,
options={id(clf): {"zipmap": False}},
)
with open("pipeline_titanic_nozipmap.onnx", "wb") as f:
f.write(model_onnx.SerializeToString())
sess = rt.InferenceSession(
"pipeline_titanic_nozipmap.onnx", providers=["CPUExecutionProvider"]
)
pred_onx = sess.run(None, inputs)
print("predict", pred_onx[0][:5])
print("predict_proba", pred_onx[1][:2])
predict [1 1 1 0 0]
predict_proba [[0.45469427 0.5453057 ]
[0.27457196 0.72542804]]
让我们检查它们是否相同。
assert_almost_equal(clf.predict_proba(X_test), pred_onx[1])
显示 ONNX 图¶
最后,让我们看看用 sklearn-onnx 转换的图。
pydot_graph = GetPydotGraph(
model_onnx.graph,
name=model_onnx.graph.name,
rankdir="TB",
node_producer=GetOpNodeProducer(
"docstring", color="yellow", fillcolor="yellow", style="filled"
),
)
pydot_graph.write_dot("pipeline_titanic.dot")
os.system("dot -O -Gdpi=300 -Tpng pipeline_titanic.dot")
image = plt.imread("pipeline_titanic.dot.png")
fig, ax = plt.subplots(figsize=(40, 20))
ax.imshow(image)
ax.axis("off")
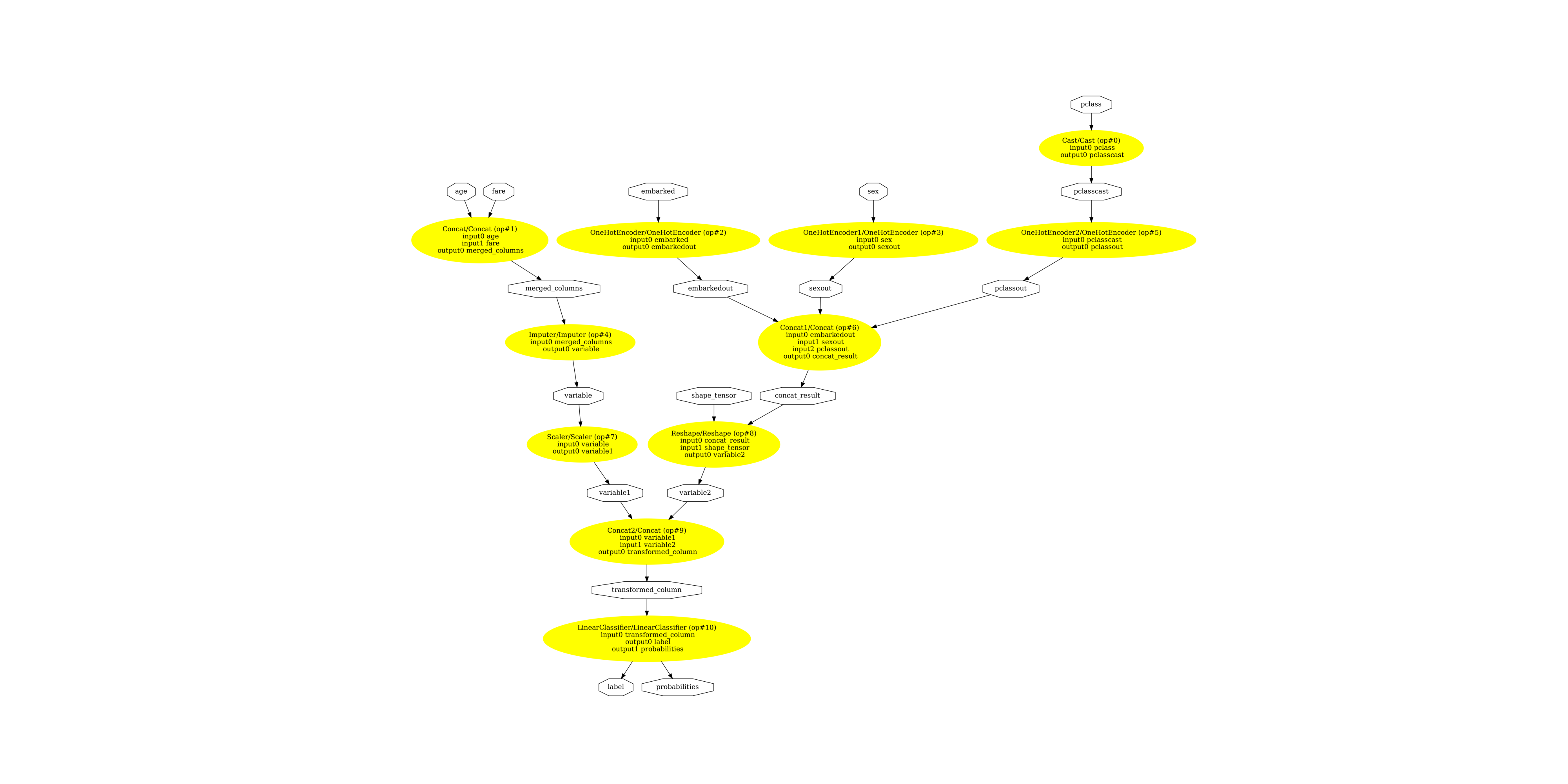
(np.float64(-0.5), np.float64(6901.5), np.float64(5287.5), np.float64(-0.5))
此示例使用的版本
print("numpy:", np.__version__)
print("scikit-learn:", sklearn.__version__)
print("onnx: ", onnx.__version__)
print("onnxruntime: ", rt.__version__)
print("skl2onnx: ", skl2onnx.__version__)
numpy: 2.2.0
scikit-learn: 1.6.0
onnx: 1.18.0
onnxruntime: 1.21.0+cu126
skl2onnx: 1.18.0
脚本总运行时间: (0 分钟 4.515 秒)