注意
转到结尾 下载完整的示例代码。
为自定义模型编写自己的转换器¶
您可能实现了自己的模型,并且显然没有此新模型的现有转换器。但这并不意味着包含它的管道的转换将无法工作。让我们看看如何做到这一点。
t-SNE 是一种有趣的转换,只能用于研究数据,因为一旦拟合就无法重现结果。这就是为什么类 TSNE 没有任何 transform 方法,只有 fit_transform。此示例提出了一种训练机器学习模型的方法,该模型近似于 t-SNE 转换器的输出。
新转换的实现¶
第一部分是关于实现的。代码非常通用,但基本上遵循以下过程来使用 X 和 y 拟合模型
t-SNE,
k 近邻,
,产生函数
最终归一化,简单缩放
并在测试集上进行预测
k 近邻,
最终归一化,简单缩放
import inspect
import os
import numpy
import onnx
from onnx.tools.net_drawer import GetPydotGraph, GetOpNodeProducer
import onnxruntime as rt
from matplotlib import offsetbox
import matplotlib.pyplot as plt
import sklearn
from sklearn.model_selection import train_test_split
from sklearn import datasets
from sklearn.base import BaseEstimator, TransformerMixin, clone
from sklearn.manifold import TSNE
from sklearn.metrics import mean_squared_error
from sklearn.neighbors import KNeighborsRegressor
from skl2onnx import update_registered_converter
import skl2onnx
from skl2onnx import convert_sklearn, get_model_alias
from skl2onnx.common._registration import get_shape_calculator
from skl2onnx.common.data_types import FloatTensorType
class PredictableTSNE(BaseEstimator, TransformerMixin):
def __init__(
self,
transformer=None,
estimator=None,
normalize=True,
keep_tsne_outputs=False,
**kwargs
):
"""
:param transformer: `TSNE` by default
:param estimator: `MLPRegressor` by default
:param normalize: normalizes the outputs, centers and normalizes
the output of the *t-SNE* and applies that same
normalization to he prediction of the estimator
:param keep_tsne_output: if True, keep raw outputs of
*TSNE* is stored in member *tsne_outputs_*
:param kwargs: sent to :meth:`set_params <mlinsights.mlmodel.
tsne_transformer.PredictableTSNE.set_params>`, see its
documentation to understand how to specify parameters
"""
TransformerMixin.__init__(self)
BaseEstimator.__init__(self)
if estimator is None:
estimator = KNeighborsRegressor()
if transformer is None:
transformer = TSNE()
self.estimator = estimator
self.transformer = transformer
self.keep_tsne_outputs = keep_tsne_outputs
if not hasattr(transformer, "fit_transform"):
raise AttributeError(
"Transformer {} does not have a 'fit_transform' "
"method.".format(type(transformer))
)
if not hasattr(estimator, "predict"):
raise AttributeError(
"Estimator {} does not have a 'predict' method.".format(type(estimator))
)
self.normalize = normalize
if kwargs:
self.set_params(**kwargs)
def fit(self, X, y, sample_weight=None):
"""
Runs a *k-means* on each class
then trains a classifier on the
extended set of features.
Parameters
----------
X : numpy array or sparse matrix of shape [n_samples,n_features]
Training data
y : numpy array of shape [n_samples, n_targets]
Target values. Will be cast to X's dtype if necessary
sample_weight : numpy array of shape [n_samples]
Individual weights for each sample
Returns
-------
self : returns an instance of self.
Attributes
----------
transformer_: trained transformeer
estimator_: trained regressor
tsne_outputs_: t-SNE outputs if *keep_tsne_outputs* is True
mean_: average of the *t-SNE* output on each dimension
inv_std_: inverse of the standard deviation of the *t-SNE*
output on each dimension
loss_: loss (*mean_squared_error*)
between the predictions and the outputs of t-SNE
"""
params = dict(y=y, sample_weight=sample_weight)
self.transformer_ = clone(self.transformer)
sig = inspect.signature(self.transformer.fit_transform)
pars = {}
for p in ["sample_weight", "y"]:
if p in sig.parameters and p in params:
pars[p] = params[p]
target = self.transformer_.fit_transform(X, **pars)
sig = inspect.signature(self.estimator.fit)
if "sample_weight" in sig.parameters:
self.estimator_ = clone(self.estimator).fit(
X, target, sample_weight=sample_weight
)
else:
self.estimator_ = clone(self.estimator).fit(X, target)
mean = target.mean(axis=0)
var = target.std(axis=0)
self.mean_ = mean
self.inv_std_ = 1.0 / var
exp = (target - mean) * self.inv_std_
got = (self.estimator_.predict(X) - mean) * self.inv_std_
self.loss_ = mean_squared_error(exp, got)
if self.keep_tsne_outputs:
self.tsne_outputs_ = exp if self.normalize else target
return self
def transform(self, X):
"""
Runs the predictions.
Parameters
----------
X : numpy array or sparse matrix of shape [n_samples,n_features]
Training data
Returns
-------
tranformed *X*
"""
pred = self.estimator_.predict(X)
if self.normalize:
pred -= self.mean_
pred *= self.inv_std_
return pred
def get_params(self, deep=True):
"""
Returns the parameters for all the embedded objects.
"""
res = {}
for k, v in self.transformer.get_params().items():
res["t_" + k] = v
for k, v in self.estimator.get_params().items():
res["e_" + k] = v
return res
def set_params(self, **values):
"""
Sets the parameters before training.
Every parameter prefixed by ``'e_'`` is an estimator
parameter, every parameter prefixed by
``t_`` is for a transformer parameter.
"""
pt, pe, pn = {}, {}, {}
for k, v in values.items():
if k.startswith("e_"):
pe[k[2:]] = v
elif k.startswith("t_"):
pt[k[2:]] = v
elif k.startswith("n_"):
pn[k[2:]] = v
else:
raise ValueError("Unexpected parameter name '{0}'.".format(k))
self.transformer.set_params(**pt)
self.estimator.set_params(**pe)
在 MNIST 上进行实验¶
让我们拟合 t-SNE…
digits = datasets.load_digits(n_class=6)
Xd = digits.data
yd = digits.target
imgs = digits.images
n_samples, n_features = Xd.shape
n_samples, n_features
X_train, X_test, y_train, y_test, imgs_train, imgs_test = train_test_split(Xd, yd, imgs)
tsne = TSNE(n_components=2, init="pca", random_state=0)
def plot_embedding(Xp, y, imgs, title=None, figsize=(12, 4)):
x_min, x_max = numpy.min(Xp, 0), numpy.max(Xp, 0)
X = (Xp - x_min) / (x_max - x_min)
fig, ax = plt.subplots(1, 2, figsize=figsize)
for i in range(X.shape[0]):
ax[0].text(
X[i, 0],
X[i, 1],
str(y[i]),
color=plt.cm.Set1(y[i] / 10.0),
fontdict={"weight": "bold", "size": 9},
)
if hasattr(offsetbox, "AnnotationBbox"):
# only print thumbnails with matplotlib > 1.0
shown_images = numpy.array([[1.0, 1.0]]) # just something big
for i in range(X.shape[0]):
dist = numpy.sum((X[i] - shown_images) ** 2, 1)
if numpy.min(dist) < 4e-3:
# don't show points that are too close
continue
shown_images = numpy.r_[shown_images, [X[i]]]
imagebox = offsetbox.AnnotationBbox(
offsetbox.OffsetImage(imgs[i], cmap=plt.cm.gray_r), X[i]
)
ax[0].add_artist(imagebox)
ax[0].set_xticks([]), ax[0].set_yticks([])
ax[1].plot(Xp[:, 0], Xp[:, 1], ".")
if title is not None:
ax[0].set_title(title)
return ax
X_train_tsne = tsne.fit_transform(X_train)
plot_embedding(X_train_tsne, y_train, imgs_train, "t-SNE embedding of the digits")
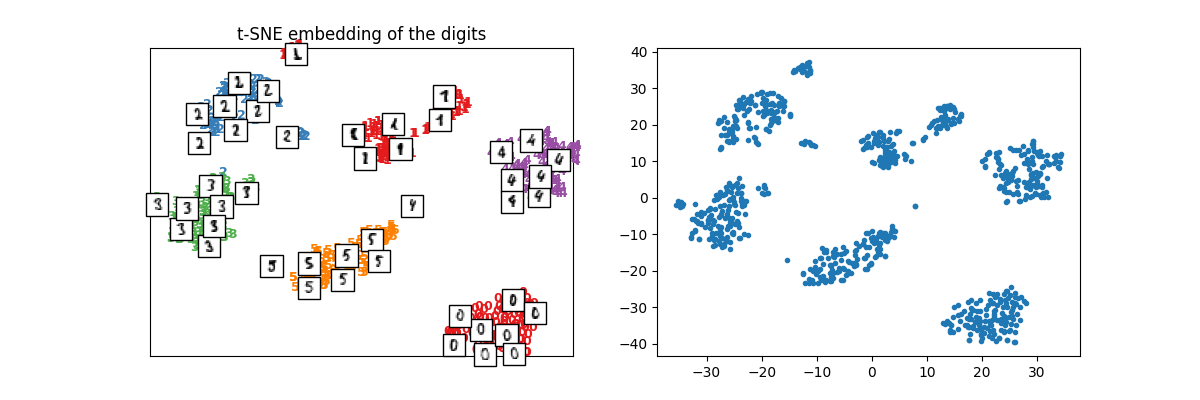
array([<Axes: title={'center': 't-SNE embedding of the digits'}>,
<Axes: >], dtype=object)
可重复的 t-SNE¶
只是为了检查它是否有效。
ptsne_knn = PredictableTSNE()
ptsne_knn.fit(X_train, y_train)
X_train_tsne2 = ptsne_knn.transform(X_train)
plot_embedding(
X_train_tsne2,
y_train,
imgs_train,
"Predictable t-SNE of the digits\n" "StandardScaler+KNeighborsRegressor",
)
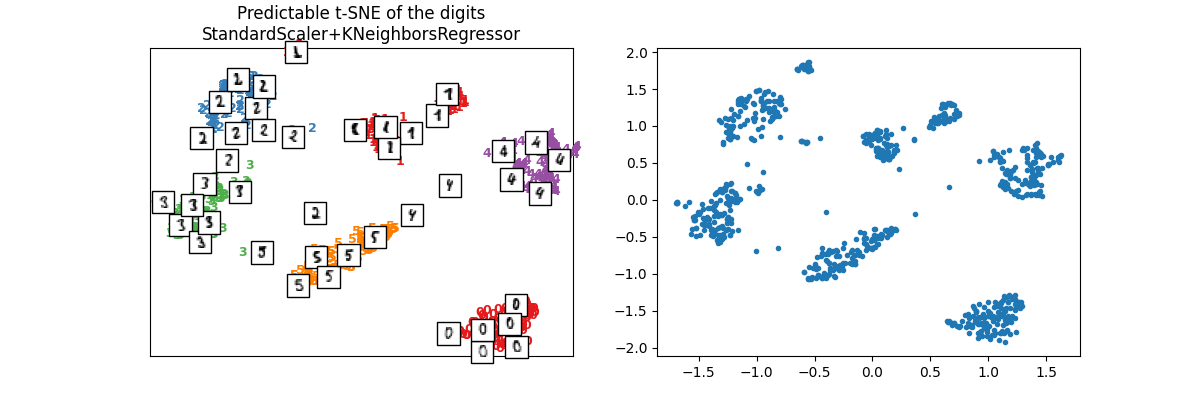
array([<Axes: title={'center': 'Predictable t-SNE of the digits\nStandardScaler+KNeighborsRegressor'}>,
<Axes: >], dtype=object)
我们在测试集上进行检查。
X_test_tsne2 = ptsne_knn.transform(X_test)
plot_embedding(
X_test_tsne2,
y_test,
imgs_test,
"Predictable t-SNE of the digits\n" "StandardScaler+KNeighborsRegressor",
)
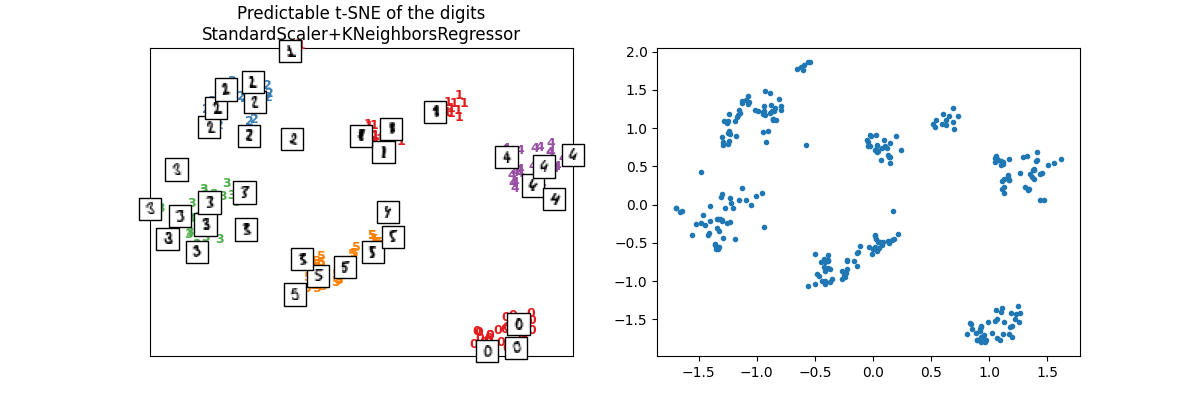
array([<Axes: title={'center': 'Predictable t-SNE of the digits\nStandardScaler+KNeighborsRegressor'}>,
<Axes: >], dtype=object)
ONNX - shape_calculator,转换器¶
现在开始专门用于 ONNX 的部分。ONNX 转换需要两个函数,一个根据输入计算输出的形状,另一个对模型进行实际转换。
def predictable_tsne_shape_calculator(operator):
input = operator.inputs[0] # inputs in ONNX graph
# output = operator.outputs[0] # output in ONNX graph
op = operator.raw_operator # scikit-learn model (mmust be fitted)
N = input.type.shape[0] # number of observations
C = op.estimator_._y.shape[1] # dimension of outputs
# new output definition
operator.outputs[0].type = FloatTensorType([N, C])
然后是转换器模型。我们重用现有的转换器。
def predictable_tsne_converter(scope, operator, container):
"""
:param scope: name space, where to keep node names, get unused new names
:param operator: operator to converter, same object as sent to
*predictable_tsne_shape_calculator*
:param container: contains the ONNX graph
"""
# input = operator.inputs[0] # input in ONNX graph
output = operator.outputs[0] # output in ONNX graph
op = operator.raw_operator # scikit-learn model (mmust be fitted)
# First step is the k nearest-neighbours,
# we reuse existing converter and declare it as local
# operator.
model = op.estimator_
alias = get_model_alias(type(model))
knn_op = scope.declare_local_operator(alias, model)
knn_op.inputs = operator.inputs
# We add an intermediate outputs.
knn_output = scope.declare_local_variable("knn_output", FloatTensorType())
knn_op.outputs.append(knn_output)
# We adjust the output of the submodel.
shape_calc = get_shape_calculator(alias)
shape_calc(knn_op)
# We add the normalizer which needs a unique node name.
name = scope.get_unique_operator_name("Scaler")
# The parameter follows the specifications of ONNX
# https://github.com/onnx/onnx/blob/main/docs/Operators-ml.md#ai.onnx.ml.Scaler
attrs = dict(
name=name,
scale=op.inv_std_.ravel().astype(numpy.float32),
offset=op.mean_.ravel().astype(numpy.float32),
)
# Let's finally add the scaler which connects the output
# of the k-nearest neighbours model to output of the whole model
# declared in ONNX graph
container.add_node(
"Scaler",
[knn_output.onnx_name],
[output.full_name],
op_domain="ai.onnx.ml",
**attrs
)
现在我们需要声明新的转换器。
update_registered_converter(
PredictableTSNE,
"CustomPredictableTSNE",
predictable_tsne_shape_calculator,
predictable_tsne_converter,
)
转换为 ONNX¶
我们只需要像任何其他模型一样调用 convert_sklearn 进行转换。
model_onnx = convert_sklearn(
ptsne_knn,
"predictable_tsne",
[("input", FloatTensorType([None, X_test.shape[1]]))],
target_opset=12,
)
# And save.
with open("predictable_tsne.onnx", "wb") as f:
f.write(model_onnx.SerializeToString())
现在我们比较预测结果。
print("ptsne_knn.tranform\n", ptsne_knn.transform(X_test[:2]))
ptsne_knn.tranform
[[-0.87237126 1.222756 ]
[ 0.13582209 0.6264854 ]]
使用 onnxruntime 进行预测。
sess = rt.InferenceSession("predictable_tsne.onnx", providers=["CPUExecutionProvider"])
pred_onx = sess.run(None, {"input": X_test[:1].astype(numpy.float32)})
print("transform", pred_onx[0])
transform [[-0.8723713 1.222756 ]]
最近邻的转换器生成一个 ONNX 图,该图不允许一次进行多次预测。让我们为第二行调用 onnxruntime。
pred_onx = sess.run(None, {"input": X_test[1:2].astype(numpy.float32)})
print("transform", pred_onx[0])
transform [[0.13582209 0.6264854 ]]
显示 ONNX 图¶
pydot_graph = GetPydotGraph(
model_onnx.graph,
name=model_onnx.graph.name,
rankdir="TB",
node_producer=GetOpNodeProducer(
"docstring", color="yellow", fillcolor="yellow", style="filled"
),
)
pydot_graph.write_dot("pipeline_tsne.dot")
os.system("dot -O -Gdpi=300 -Tpng pipeline_tsne.dot")
image = plt.imread("pipeline_tsne.dot.png")
fig, ax = plt.subplots(figsize=(40, 20))
ax.imshow(image)
ax.axis("off")
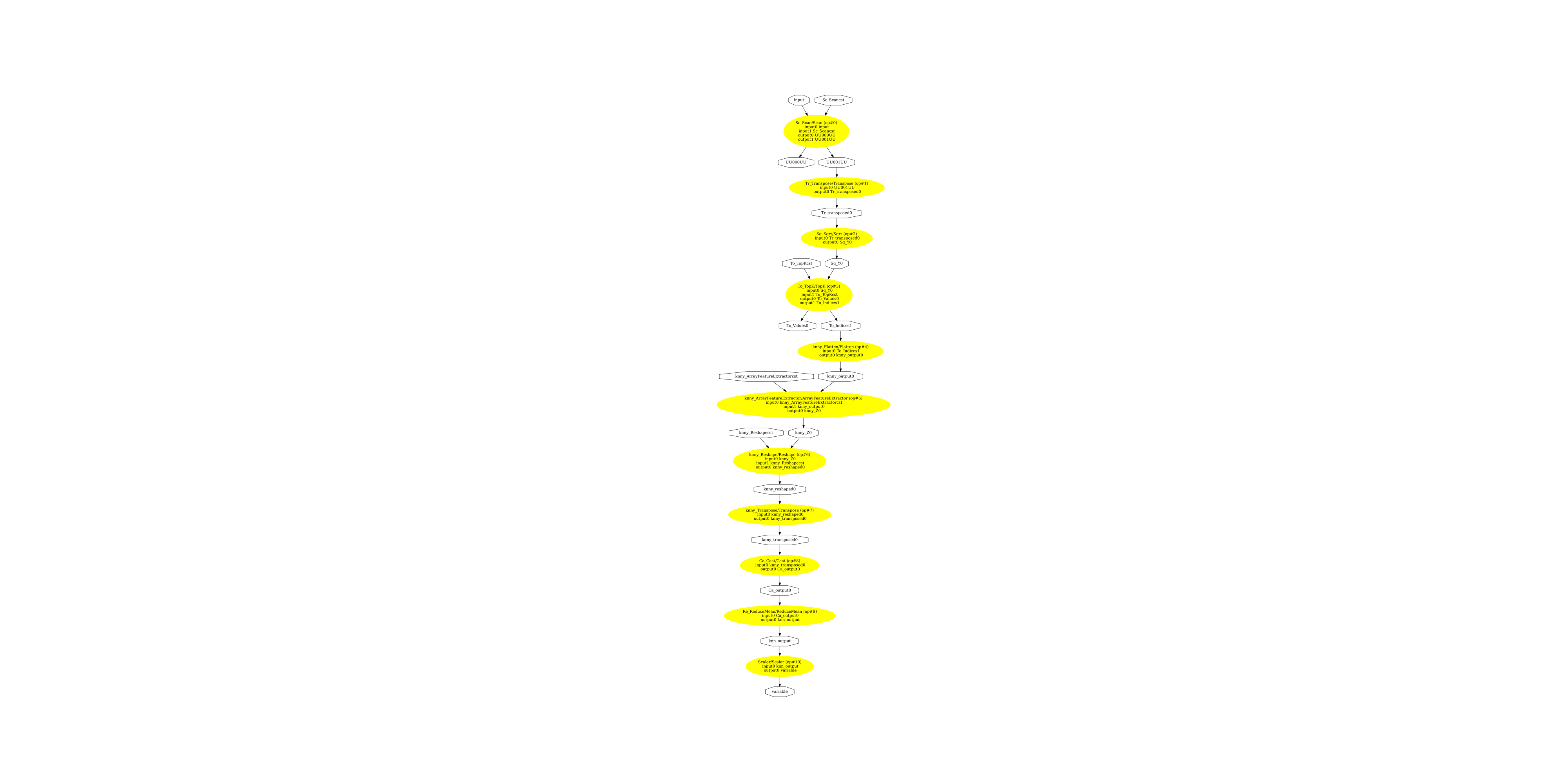
(-0.5, 2643.5, 9099.5, -0.5)
此示例使用的版本
print("numpy:", numpy.__version__)
print("scikit-learn:", sklearn.__version__)
print("onnx: ", onnx.__version__)
print("onnxruntime: ", rt.__version__)
print("skl2onnx: ", skl2onnx.__version__)
numpy: 1.26.4
scikit-learn: 1.6.dev0
onnx: 1.17.0
onnxruntime: 1.18.0+cu118
skl2onnx: 1.17.0
脚本的总运行时间:(0 分 10.217 秒)